AI in Education: Theory and Practice
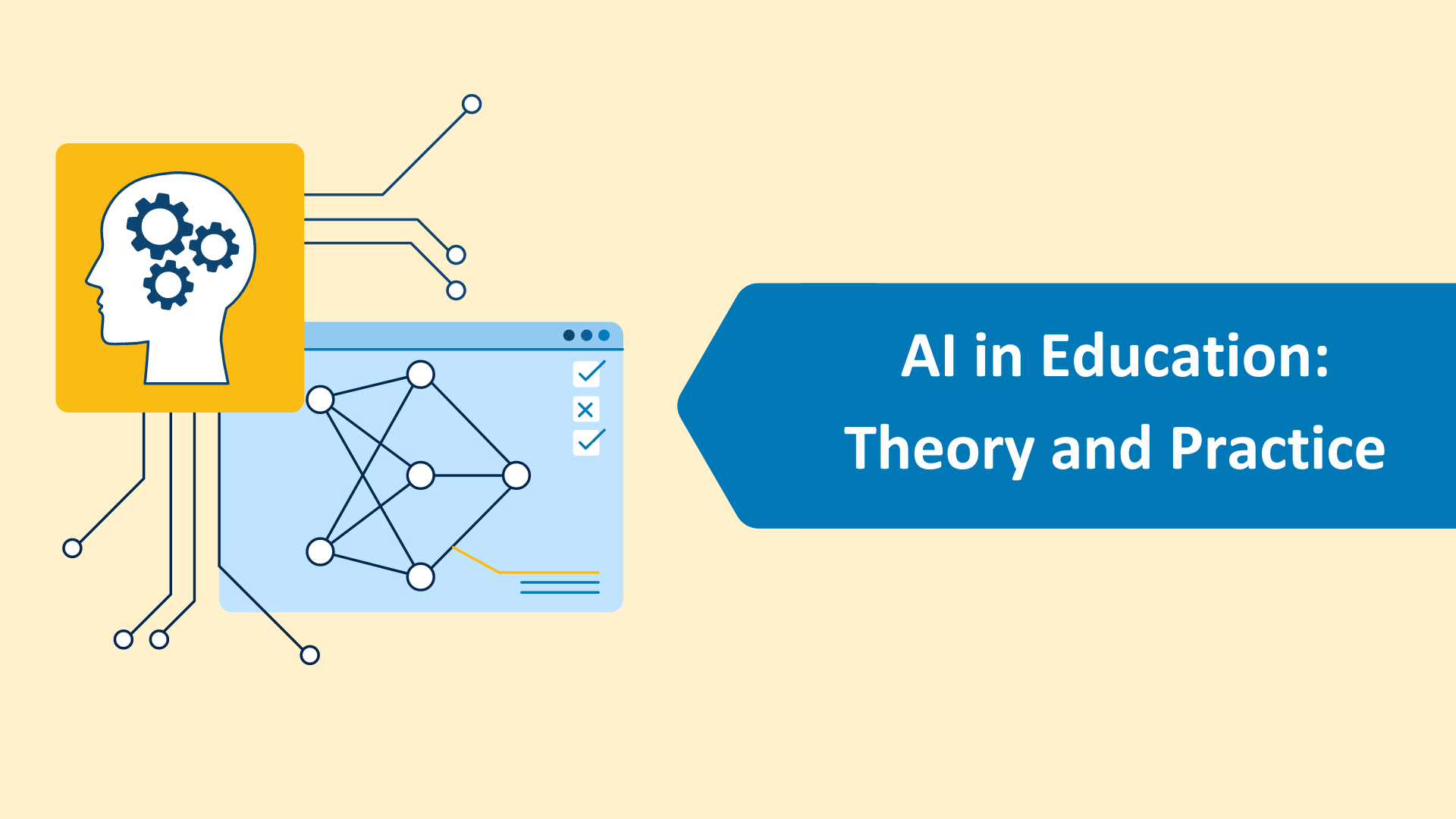
Overview
Content
The benefits of integrating AI into STEM lessons and projects
Promoting computational thinking and creativity
Preparing for future careers
Fostering teamwork and inclusion
Addressing social, ethical and ecological implications
Theoretical approach to implementing AI in an educational context
Pedagogical considerations
Putting theory into practice: the AI in STEM teaching units
As artificial intelligence becomes more and more present in our daily lives, both in the workplace and in leisure spaces as well as in the education sector, it is important for students to familiarise themselves with its concepts and applications from the earliest levels of schooling. Teaching about AI in school provides an engaging opportunity for students to explore and apply advanced technology concepts in practical and creative projects, while offering a wide range of pedagogical benefits.
Author: Liliana Fernandes
The benefits of integrating AI into STEM lessons and projects
Promoting computational thinking and creativity
Learning-by-doing methodologies such as Project-Based Learning (PBL) and Inquiry-Based Learning (IBL) in STEAM provide students with an environment where they can experiment and apply theoretical knowledge. The integration of AI tools in schools will offer an additional dimension, allowing students to acquire skills involving machine learning, natural language processing, and computational thinking, among others. This new premise will help them integrate theoretical content into solving real-world problems by creating interactive projects, testing artificial intelligence algorithms and having the opportunity to observe how these tools work in practice. In addition, this approach, by offering various options for exploring innovative ideas, will have a significant impact on students' creativity.
Experience AI: Experience AI is an educational programme that offers resources on artificial intelligence and machine learning for teachers and students aged 11–14. Developed in collaboration by the Raspberry Pi Foundation and Google DeepMind, the programme supports teachers in the exciting and fast-moving area of AI, and gets young people passionate about the subject. Includes free lesson sets complete with lesson plans, presentations, videos, and worksheets. (Registration required)
Day of AI curriculum: Developed by leading faculty and educators from MIT RAISE (Responsible AI for Social Empowerment and Education), each curriculum features a series of 30-60 minute lessons that engage kids in creative discovery, discussion, and critical thinking as they learn the fundamentals of AI, investigate its societal impacts, and bring grade-relevant applications of artificial intelligence to life through hands-on activities that are accessible to all, even for those with no computer science or technical background. (Registration required)
Code.org AI curricula: Includes short "How AI works" videos and free lessons/teaching units for all age levels on a range of topics from "AI for Oceans" to "AI Ethics"
Machine Learning for Kids UK: A free tool introducing machine learning by providing hands-on experiences for training machine learning systems and building things with them. It provides an easy-to-use guided environment for training machine learning models to recognise text, numbers, images, or sounds.
I Am AI: Resources to read, build, make music, experiment and play with AI.
Preparing for future careers
The integration of these tools also makes it possible to develop technical skills, from understanding the fundamentals of AI to the practical application of this knowledge, as well as coding, data analysis, algorithm design and systems engineering skills that are essential for the future labour market, thus preparing students for future careers in technology-related and creative fields.
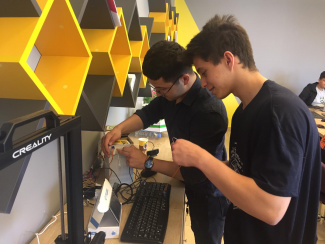
Fostering teamwork and inclusion
Creating AI projects in class requires an interdisciplinary approach, involving knowledge of maths, engineering, coding, science and even areas such as ethics or philosophy, and is often based on team collaboration and problem-solving, which encourages students to work together, share knowledge and overcome complex challenges.
Furthermore, the integration of AI into active learning environments has the potential to play a transformative role in promoting inclusive education. By enabling accessibility, personalisation of learning, assisted learning, social inclusion and collaboration, as well as, at a later stage, inclusive assessment, AI can create a more equitable pedagogical environment where all students have the opportunity to evolve consistently.
AI can be used to develop accessible technologies such as voice recognition systems and automatic translation APPs or devices, enabling students with different needs and levels of competence to interact effectively with educational tools and resources and empowering them to actively participate in learning. AI-assisted learning is another strand through which inclusion is promoted; AI-based virtual assistants can offer students permanent personalised support during the creation and problem-solving process, providing step-by-step guidance, useful tips and immediate feedback suited to their level of competence and learning style.
In addition to other advantages such as supporting more inclusive assessment processes, we might also mention that the introduction of AI in makerspaces and classrooms even contributes to reducing the gender gap in STEAM areas. In February 2016, at a TED talk, Reshma Saujani, founder of the “Girls Who Code” programme, said that the large deficit of women in STEAM areas was due to the fact that we are educating boys to be brave and girls to be perfect, reaffirming the urgency of changing the paradigm.[1] With the introduction of coding and AI, this gap can be narrowed, as the methodology requires perseverance and shows that mistakes are not “fatal”; on the contrary, it demonstrates that success is a path of corrected mistakes, helping to demystify the idea that girls have to be perfect.
Addressing social, ethical and ecological implications
Finally, students' social and ethical awareness is developed, as working with AI also involves discussing and debating ethical and social issues related to this technology. Through understanding and applying AI, students will explore topics such as privacy, data protection and the impact of AI on society as well as the need to make the technology more sustainable.
The topic of sustainability, for example, offers the opportunity to shed light on the massive energy consumption of large AI models on the one hand and to learn about specialised AI applications that can play a positive role in environmental protection on the other. Critical reflection on the handling of personal data and copyright-protected works when training large AI models as well as on challenges posed by deepfakes and other ill-intended manipulations can also be part of a classroom discussion.
“Ethics guidelines for trustworthy AI”, publication of the Directorate-General for Communication Netzworks, Content and Technology of the European Commission, 2019, and “Ethical guidelines on the use of artificial intelligence and data in teaching and learning for educators”, publication of the Directorate-General for Education, Youth, Sport and Culture of the European Commission, 2022.
"Artificial intelligence in education: challenges and opportunities for sustainable development", UNESCO Working Paper on Education Policies, 2019, full text available online.
"Ethical AI for Teaching and Learning", Center for Teaching Innovation, Cornell University.
"The Green Dilemma: Can AI Fulfil Its Potential Without Harming the Environment?", Earth.org, July 2023.
A Pidgeon's Tale. A Comic Essay on Artificial Intelligence and Sustainability, by Dr. Julia Schneider, illustrated by Pauline Cremer (retrieved on July 16, 2024).
Theoretical approach to implementing AI in an educational context
To successfully implement the integration of AI tools into school, it is essential to ensure that the right resources and support are made available to students and teachers, including access to relevant hardware and software, teacher training, partnerships with AI experts and the integration of AI projects into the school curriculum.
To help establish a guideline for implementing AI in an educational context, Touretzky et al (2019)[2] proposed what they call the “five big ideas in AI”: perception, representation and reasoning, learning, natural interaction and social impact. These five big ideas provide a broad basis for exploring the fundamental concepts in AI and promoting AI literacy among students. By understanding these principles, students can develop a deeper understanding of how AI works, as well as its implications for different aspects of society.
In this approach, perception refers to the ability of AI systems to recognise and understand the world around them in the same way as humans, which involves processing sensory data such as images, audio and/or text to extract meaningful information. For example, in computer vision, AI algorithms can be trained to recognise objects, faces or patterns in images, using techniques such as convolutional neural networks. In natural language processing, AI systems can analyse and understand the meaning of written or spoken text, enabling automatic translation or the extraction of information from large volumes of text. This ability to perceive is fundamental to many AI applications, from voice recognition systems in virtual assistants to computer vision systems in autonomous vehicles.
The second idea proposed refers to representation and reasoning, which are essential for AI systems to be able to organise knowledge in a meaningful way and make autonomous decisions based on that knowledge. In symbolic artificial intelligence, systems represent knowledge using symbols and logical rules, enabling deductive reasoning. For example, in expert systems, knowledge is represented as a set of production rules, which are used to infer new information from input data. In deep learning, on the other hand, systems learn high-level representations of data through layers of processing, enabling inductive reasoning in tasks such as pattern recognition or prediction. This idea is central to the development of AI systems capable of solving complex problems and making autonomous decisions.
Learning is one of the most powerful capabilities in AI, allowing systems to improve their performance in specific tasks based on experience. There are different types of learning in AI, including supervised, unsupervised and reinforcement learning. In supervised learning, systems are trained on labelled input-output pairs, allowing them to learn to associate inputs with desired outputs. For example, in image recognition, a system can be trained on a set of labelled images to recognise specific objects. In unsupervised learning, systems learn patterns and structures in the data without external guidance, allowing for the discovery of insights and groupings. In reinforcement learning, systems learn to make decisions and take actions in an environment to maximise a reward, using techniques such as Q-learning or genetic algorithms. This ability to learn from experience is fundamental to the development of AI systems that can adapt and improve over time.
Natural interaction, the fourth idea proposed, presupposes security and ethics as critical considerations in the development and use of artificial intelligence, given its growing influence on various aspects of society. Security issues include protection against cyber attacks and guaranteeing the integrity and reliability of AI systems. Ethical issues involve concerns about algorithmic bias, data privacy, fairness and transparency. AI algorithms can reflect and even amplify existing biases in training data, leading to discriminatory decisions in areas such as hiring, business transactions or criminal justice. What's more, the use of personal data in AI systems raises concerns about privacy and the protection of individual rights. It is crucial to address these safety and ethical issues to ensure that AI is developed and used in a responsible and inclusive manner.
Finally, the social and economic impact of AI is broad and far-reaching, affecting areas such as the labour market, education, health or politics. AI-driven automation is transforming the nature of work, with potential effects on employment, wages and income distribution.
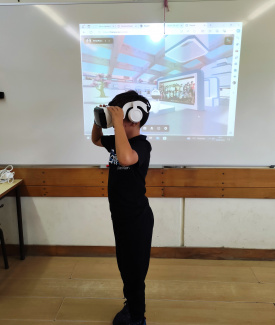
Pedagogical considerations
In a pedagogical environment, we cannot neglect the assessment of students' achievements. The question then arises: how do we assess learning in environments that integrate AI? As there is no formal assessment framework for AI in education yet, we suggest looking at Brennan & Resnick's (2012)[3] proposal, which proposes three approaches to assessing the development of computational thinking in young people involved in design activities with Scratch software: project portfolio analysis; interviews, where students are asked about the process of developing their projects; and the use of design scenarios, in which students are presented with projects created by other Scratch users, and asked to reflect on different aspects of the projects, such as their length, problem-solving and remixing.
Putting theory into practice: the AI in STEM teaching units
On this website, we propose some project and teaching ideas to implement in a school environment, integrating active teaching methodologies, Project-Based Learning, the STEAM approach and artificial intelligence. From primary to secondary, whether you are a beginner or an expert, you will find different proposals and approaches, adaptable to your own teaching reality. Take first steps with some generative AI tools and data analysis to learn and teach about Big Data, machine learning and citizen science. Use role play and a VR environment to familiarise your students with basic coding and robotics involving an AI-based recycling application. Inspire your more advanced students to design, code and build a smart recycling system of their own or create digital educational resources for different STEM subjects using AI and machine learning. And to dive deeper into the topic of artificial neural networks and some of their most common applications, explore our comprehensive interactive course on "Machine Learning in School".
Saujani, Reshma, "Teach Girls Bravery, not Perfection", TED Talk, 2016.
Touretzky, D., Gardner-McCune, C., Martin, F., & Seehorn, D., "Envisioning AI for K-12: What should every child know about AI?". In: Proceedings of the AAAI conference on artificial intelligence. July 2019, Vol. 33, No. 01, pp. 9795-9799. Available online at: https://www.researchgate.net/publication/335221360_Envisioning_AI_for_K-12_What_Should_Every_Child_Know_about_AI
Brennan, K., & Resnick, M., "New frameworks for studying and assessing the development of computational thinking." In: Proceedings of the 2012 annual meeting of the American educational research association, Vancouver, Canada. April 2012, Vol. 1, p. 25. Available online at: https://www.media.mit.edu/publications/new-frameworks-for-studying-and-assessing-the-development-of-computational-thinking/
Share this page